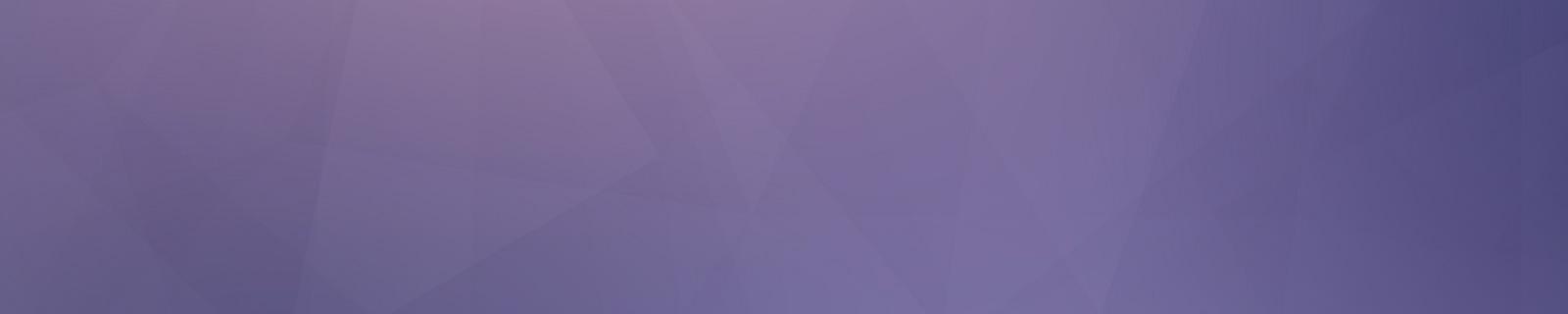
Comparative Effectiveness & Implementation Research Training Program Course Descriptions
In NYU Grossman School of Medicine’s Comparative Effectiveness and Implementation Research Training Program, training in both programs offered—the MS in clinical investigation, with a concentration in comparative effectiveness and implementation research; and advanced certificate—is built around five foundational courses.
Early Summer Courses
The early summer courses are selectives. Students choose one of the following courses.
Introduction to Dissemination and Implementation Science Research (3 Credits)
This course provides a comprehensive introduction to dissemination and implementation (D&I) science research to enable students to develop core competencies in D&I science. The course enhances students’ ability to conceptualize and think through D&I research problems and apply theory and employ approaches to improve implementation outcomes with increasing independence. Students complete outside readings throughout the course and are expected to successfully apply an implementation science framework to a prepared research question by the end of the course.
Health Communications: Applied Methods for Recruiting and Retaining Participants in Clinical Trials (3 Credits)
The importance of efficiently and ethically recruiting and retaining participants in clinical trials has gained recognition by the NIH, FDA, and other groups in recent years, particularly with regard to women, minorities, and older adults. This 5-day course will cover contemporary methods in recruiting and retaining participants across behavioral and health condition contexts. Specifically, students will learn about topics including but not limited to: best practices in recruitment and retention, tools to determine whether your participant pool is feasible, how to write thorough recruitment sections for grants, how to train study team members to recruit effectively, and the growing use of recruitment tracking dashboards by funders and academic institutions. Emerging issues such as how, if at all, to use social media for recruitment and considerations for recruiting vulnerable populations (for example, pregnant women, or those with substance use disorders) will also be addressed. This course will provide a combination of lecture-based didactic training, interactive exercises designed to advance students' individual research projects, and cases studies from the news and academic journals. Active participation is expected and required.
Fall Courses
Certificate students have a third selective course for the fall: Advanced Methods in Observational Data Analysis, Biostatistics Part III. Master’s students are required to complete all fall courses.
Meta-Analyses and Systematic Reviews (3 Credits)
This course trains students to conduct a systematic literature review, considered by many investigators to be the highest level of evidence for answering clinical questions. This graduate‐level course is comprised of didactic classroom sessions and lectures on the topic as well as the hands-on conducting of a systematic review on a topic. Students are taught how to perform each step in a review and apply it to a topic of interest that they either choose at the beginning of the class, or have provided to them. Lab sessions focus on practical aspects of meta-analysis. Analyses are performed using RevMan software, which is available as a free download. At the completion of this course, students should be able to formulate key questions for a systematic review, organize a literature search, identify which literature databases to search, abstract relevant information from studies in a systematic manner, rate the scientific quality of each study, create evidence tables and summary tables, summarize the studies’ findings, and interpret findings. The final deliverable for the course will be a systematic literature review presentation.
Economic Evaluation in Health and Medicine (3 Credits)
The purpose of this course is to introduce concepts and techniques used in the economic evaluation of healthcare interventions and develop a specific research question; apply best practices of model building; and conduct analysis and interpret results. This course focuses on the methods of cost-effectiveness analysis. Decision analysis in general, and cost-effectiveness analysis in particular, is an approach to help decision makers systematically and simultaneously compare a full range of options, apply all relevant evidence (what is known and unknown), consider consequences of action (or inaction), and incorporate patient preferences. This course provides an understanding of the foundations of decision analysis and cost-effectiveness analysis, with sufficient detail regarding the mechanics and methodologies to prepare students to both interpret and critique the literature of cost-effectiveness analysis and construct these analyses themselves.
Advanced Methods in Observational Data Analysis, Biostatistics Part III (3 credits)
This course builds on prior training in introductory biostatistics and epidemiology to extend understanding of core concepts and methods by providing applied training in the conduct of secondary data analysis studies. Using an existing data source, students identify a research question; define a causal model, specific aims, and hypotheses; gain experience in management and conditioning of the data; conduct stratified analyses to assess effect modification and confounding; implement the backward elimination method of model building using logistic regression to obtain multivariable results; and interpret results with respect to the strength and precision of estimates, selection and information bias, and confoundedness, missingness, and generalizability. Students are trained in and use SAS or STATA for all data cleaning, conditioning, and analysis. In addition, advanced topics in statistics, such as the use of propensity scores to address confounding and use of mixed models for clustered data, are introduced.