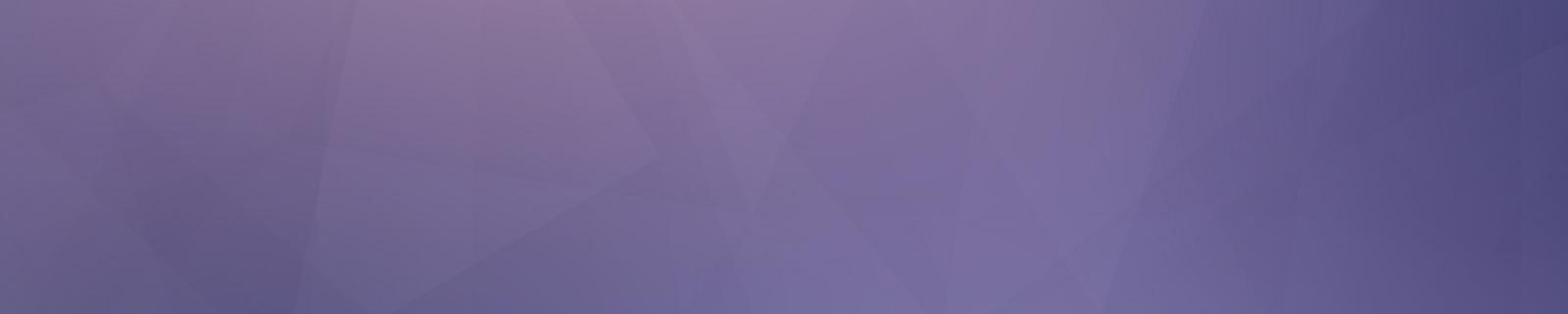
Stress, Trauma & Resilience Research
Researchers, clinicians, and educational specialists at NYU Langone’s Department of Child and Adolescent Psychiatry study stress, trauma, and resilience. Our aims are to develop new treatments for children who have experienced trauma, support resilience among children and their families, and prevent the development of traumatic stress responses.
We conduct translational research to better understand the basic processes that underlie the way adverse experiences affect brain development, create new methods to identify risks associated with traumatic stress, and develop novel treatments, including large-scale community interventions.
Our researchers work closely with clinicians who provide various evidence-based therapies, including child–parent psychotherapy, trauma-focused cognitive behavioral therapy, and trauma systems therapy.
Stress, Trauma, and Resilience Research Laboratories and Programs
We collaborate with clinical investigators on the Stress, Trauma, and Resilience Treatment Service at the Child Study Center and the Center for Child and Family Resilience at the Sala Institute for Child and Family Centered Care, all part of Hassenfeld Children’s Hospital at NYU Langone.
Glenn Saxe Laboratory
Researchers in the laboratory of Glenn Saxe, MD, study the complex needs of children who have experienced trauma and seek to improve the standard of mental health services available to them.
We also study the social, developmental, and biobehavioral processes that influence the expression of traumatic stress in children in their social environments and the systems that serve them. The lab includes studies of the application of advanced computational methods to uncover possible etiological factors for child traumatic stress, implementation and evaluation of innovative practices in typical care settings, and dissemination of practices shown to have potential for large-scale impact.
Dr. Saxe also leads a team of child and adolescent psychiatry faculty who provide training on trauma systems therapy, a trauma-focused intervention he co-developed to assess and treat children with traumatic stress.
Trauma-Informed Juvenile Justice Program
In the Trauma-Informed Juvenile Justice Program, we focus on addressing the effects of trauma on youth in the juvenile justice system. An estimated 90 percent of juveniles in secure detention have experienced trauma—from physical and sexual abuse to community violence—often leading to anger, depression, and challenging behaviors.
The program, based at NYC Health + Hospitals/Bellevue and funded by the federal Substance Abuse and Mental Health Services Administration, is a Category III (community practice) center of the National Child Traumatic Stress Network. We collaborate with the Division of Youth and Family Justice at the New York City Administration for Children’s Services.
The program’s aims are to create high-quality screening tools to identify a child’s trauma history and its impact on his or her functioning; develop effective training programs for corrections officers; put in place intervention programs to address traumatic stress problems for youth in detention; create consultation programs to help detention facility administrators address the needs of traumatized children; and develop legal advocacy programs to educate judges and others in the legal system about the link between traumatic stress and violent or criminal behavior.
Finding Effective Approaches to Childhood Stress and Trauma
Read More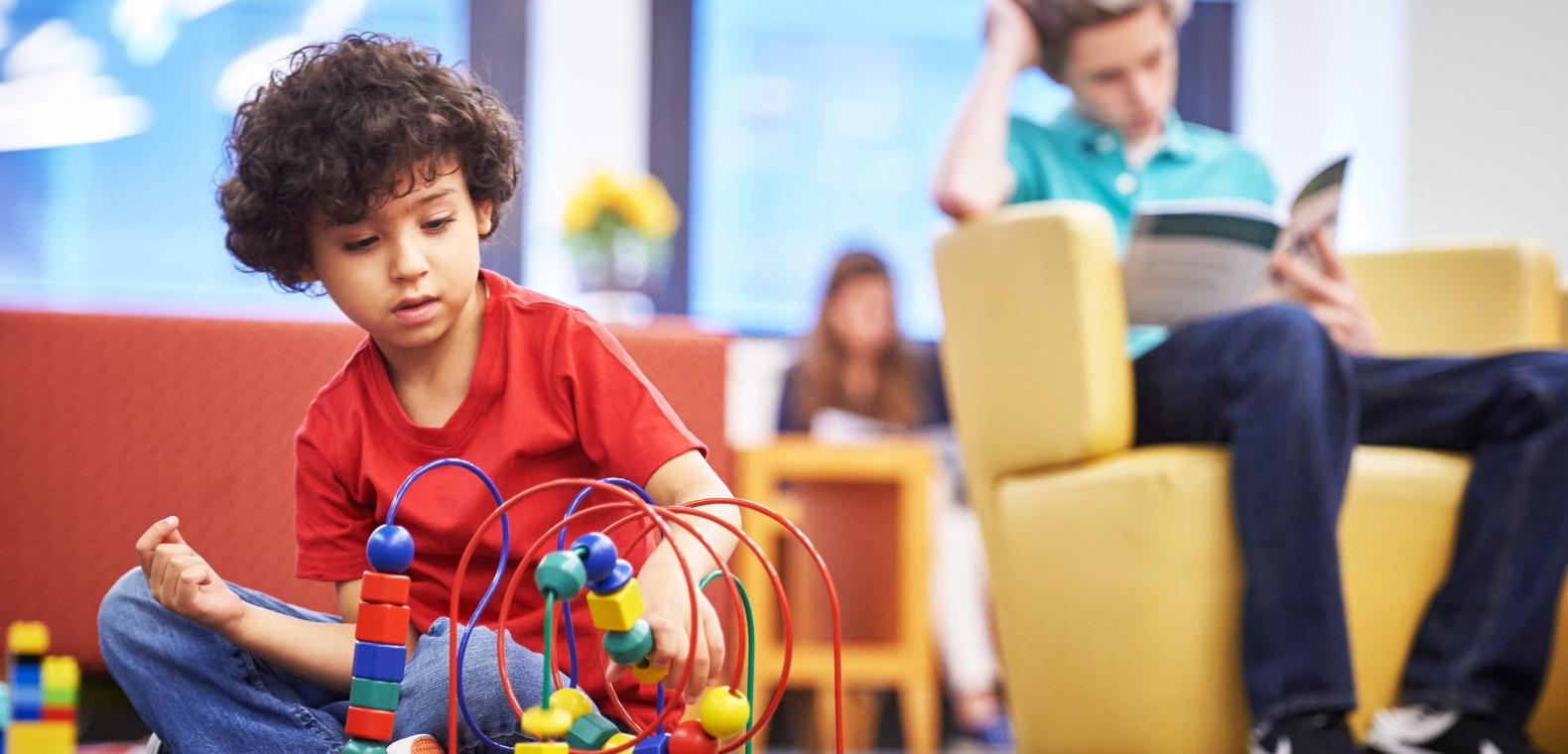