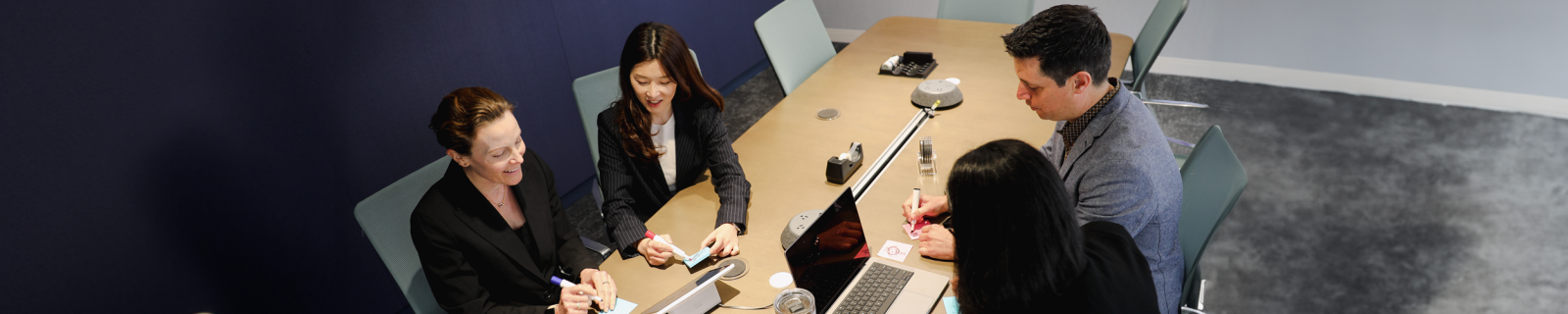
Healthcare Innovation Bridging Research, Informatics & Design Lab Projects
The Healthcare Innovation Bridging Research, Informatics, and Design (HiBRID) Lab collaborates with researchers across NYU Langone on a variety of research and operational projects. We aim to improve healthcare delivery and patient care by supporting user-centered design in digital health applications and electronic health record (EHR) innovations.
Our unique interdisciplinary research group currently manages a portfolio of seven federally sponsored grants and multiple enterprise digital innovation initiatives. We represent core members of critical digital innovation teams at NYU Langone including Clinical Decision Support, FuturePractice, Patient Digital Experience, and Clinical Digital Experience.
Learn more about our current projects below.
HiBRID Co-Pilot Study
This project aims to build and design AI copilots for different use cases to implement into a digital health practice, with the goal of increasing innovation, alleviate administrative burden, and enhance research efficiency.
Engage-Rx
Engage-Rx is a web-based clinical decision support (CDS) tool for management of chronic diseases (starting with hypertension (HTN)) through patient engagement and automated algorithmic clinical decision support. This project aims to link Engage-Rx and Eureka Research Platform to create a singular EHR-connected scalable and modifiable technology platform that delivers integrated patient-and clinician-facing interventions.
Digital Diabetes Prevention Integration Tool (Noom-dDPP)
This study, Evaluation of an Automated Physician-Directed Messaging on Patient Engagement in the Digital Diabetes Prevention Program, aims to understand the effectiveness of a personalized SMS integration tool (PAMS) on patient engagement within a digital diabetes prevention program (dDPP) through measurement of weight, A1c, and Noom use. The project leverages a National Institutes of Health (NIH)–funded prototype integration tool with the goal of conducting a large-scale hybrid effectiveness and implementation study.
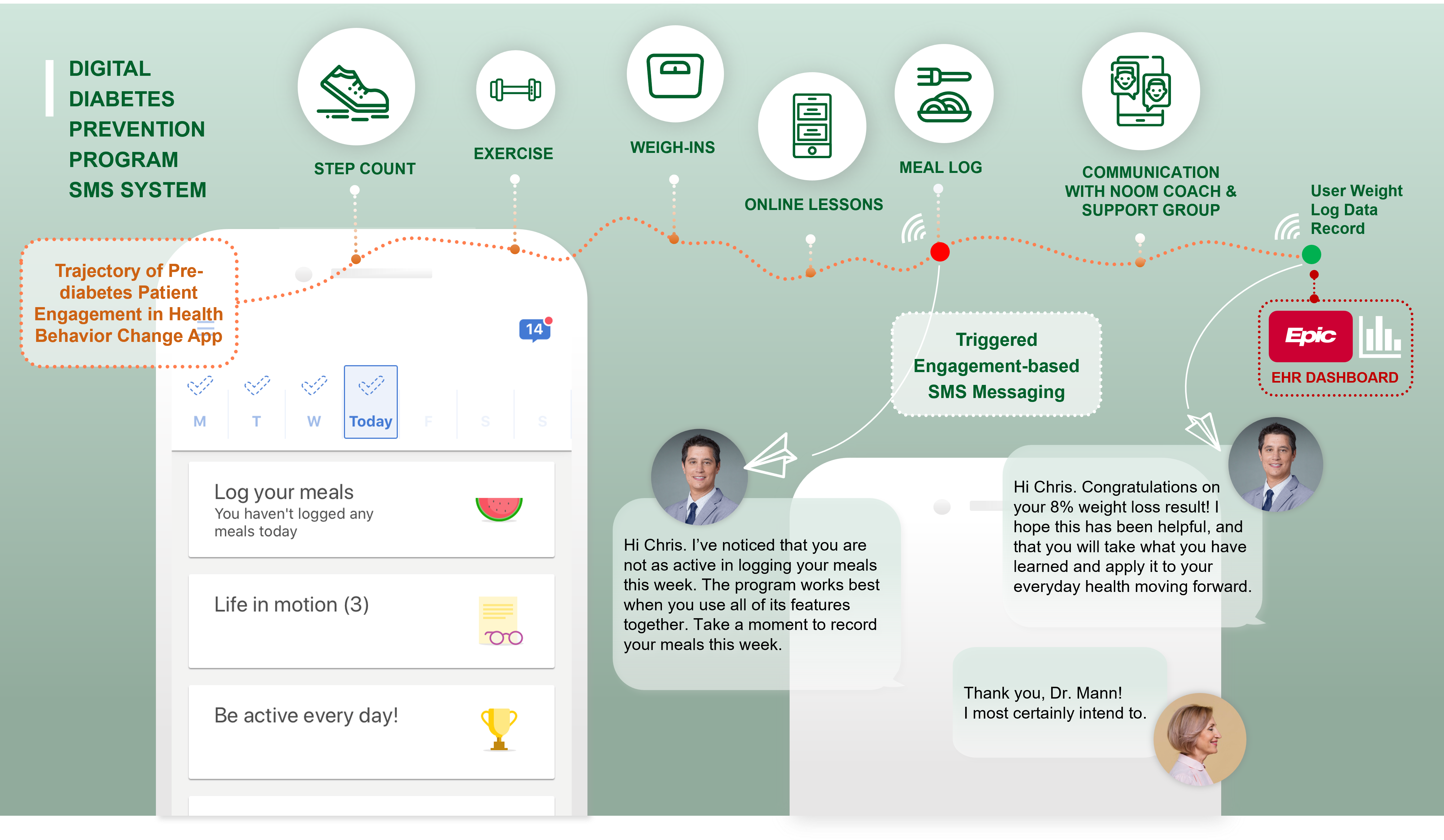
Effects of Nurse-Driven Integrated Clinical Prediction Tool on Antibiotic Prescribing (iCPR3)
This study, Decreasing Antibiotic Prescribing in Acute Respiratory Infections Through Nurse-Driven Clinical Decision Support, evaluates the effects of a novel integrated clinical decision prediction tool, iCPR3, on antibiotic prescription patterns of nurses for acute respiratory infections (ARIs). The intervention is an EHR-integrated risk calculator and order set to help guide appropriate, evidence-based antibiotic prescriptions for patients presenting with ARI symptoms.
Team Care to Address Medication Adherence Leveraging EHR Technology (TEAMLET)
This project, Addressing Antihypertensive Medication Adherence Through EHR-enabled Teamlets in Primary Care, involves pragmatic, type I hybrid comparative effectiveness–implementation study. TEAMLET aims to evaluate if an Epic-based intervention (pharmacy fill data, EHR-based clinical decision support, and health coaching by primary care providers) can improve medication adherence and blood pressure control in the primary care setting.
Remote Patient Monitoring Playbook
This project includes characterizing baseline remote patient monitoring (RPM) workflows and pain points across different specialties, designing and testing solutions to address these problems in tandem with the HiBRID Lab, NYU Langone’s Medical Center Information Technology (MCIT), and clinical stakeholders, and creating an internal “playbook” that outlines a comprehensive strategy for using RPM across NYU Langone clinics.
Lung Cancer Screening Clinical Decision Support Tool for Shared Decision-Making
This project aims to improve lung cancer screening at a large scale through clinical decision support (CDS) using shared decision-making (SDM) with the University of Utah. In order to achieve our goal, we will be utilizing a Decision Precision+ app using the “SMART on FHIR” interoperability standard for lung cancer screening.
EHR Nudges: Optimizing a Clinical Decision Support System for Evidence-Based Statin Medication Prescribing to Reduce the Risk of Cardiovascular Disease
This project aims to develop and optimize a clinical decision support system, including several electronic health record nudges, to increase guideline-concordant statin prescribing.
Nudging Provider Adoption of Clinical Decision Support: Implementation of an EHR-Agnostic Pulmonary Embolism Risk Prediction Tool
This project aims to examine the imapct of a theory-informed "nudge" implementation strategy on the adoption of guideline-concordant CT ordering for PE in 10 EDs, and to determine the impact of the nudge implementation strategy on identified behavioral barries to adoption of guideline-concordant CT ordering.
AI in Anti-Obesity Medication Management
This project aims to develop an AI-driven intervention to improve the management of anti-obesity medications (GLP-1).
Prototype for GenAI-powered Remote Patient Monitoring (RPM) Assistant for Hypertension
This project aims to use GenAI to automate key patient and provider experiences to improve efficiency/sustainability of a Hypertension Management Remote Patient Monitoring (RPM) and intends to improve the RPM program by delivering more personalized patient journeys, improving clinician efficiencies, and establishing sustainability for RPM program.
Alta 2.0: Partners in Control
This project, funded by the National Institute on Minority Health and Health Disparities, aims to develop and evaluate the effectiveness of remote patient monitoring (RPM)-enabled community health workers (CHWs) in improving hypertension management among Latine patients. It focuses on developing and evaluating the effectiveness of training modules and electronic health record tools for CHWs to improve the use of remote patient monitoring to improve blood pressure control among this population.
iMatter2.0
This project utilizes an AI-driven approach to supercharge a novel digital patient-reported outcomes tool for diabetes management and examine the associations between patients’ PRO responses and HbA1c reduction.
Advancing the Development of Collaborative Research Networks to Enhance Aging Research Value
This research study is funded by the National Institute on Aging (NIA) and aims to determine factors contributing to the development of successful research collaboration networks to support increased interdisciplinary collaboration and diversity in aging research.
National Science Foundation Digital Worker
This research is funded by the National Science Foundation (NSF) and studies how to best bring inclusive tech into clinical settings, empower healthcare workers to take advantage of data-driven research, and improve health outcomes for patients. This study collaborates with NYU Tandon School of Engineering and NYU Stern School of Business.