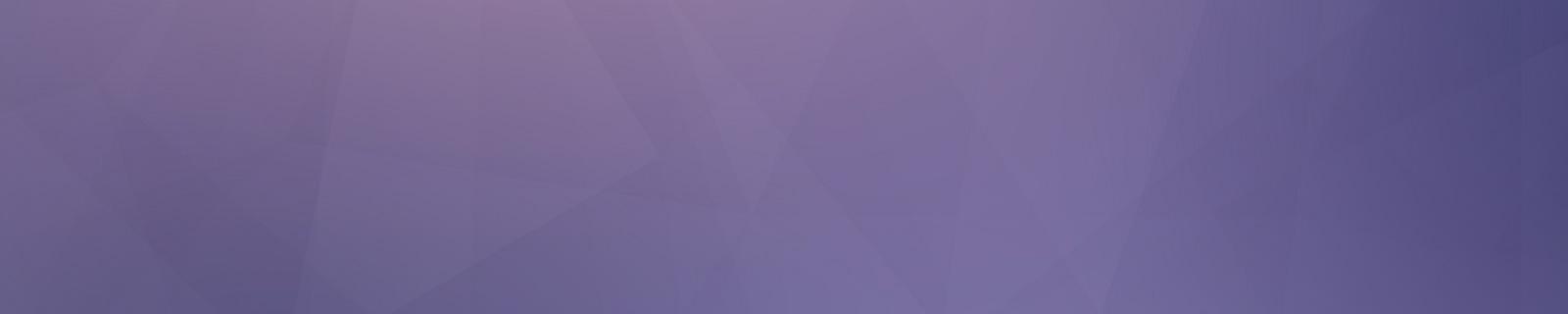
Predictive Analytics & Machine Learning
The Predictive Analytics Unit in the MCIT Department of Health Informatics uses data and modeling to predict health outcomes across NYU Langone. Our goal is to help clinicians and other staff in our health system make important clinical decisions in real time, increase operational efficiency, and develop as a learning healthcare system.
Led by Yindalon Aphinyanaphongs, MD, PhD, we develop, test, implement, and evaluate predictive models, many using artificial intelligence (AI) methods, to improve care. Clinical models currently in use include prediction of patients at risk of deterioration, patients who have sepsis, and patients at high risk of near-term mortality. Operational models currently in use include prediction of no-show visits and identification of patients with active diseases that have not been recorded in the electronic health record.
We also use our electronic health record data combined with AI methods to forecast and prevent onset of disease. Conditions of interest include dialysis and kidney failure, congestive heart failure, stroke, breast cancer, prostate cancer, lung cancer, type 2 diabetes, hospital-acquired infections, adverse drug interactions, emergency department visits, and rehospitalization, among others.
Our group routinely offers the following services:
- provide internal capacity for predictive modeling at NYU Langone to facilitate real-time clinical decision-making, increase operational efficiency, and build foundation for a learning healthcare system
- lead specific projects from inception through development, deployment, and evaluation
- provide education and professional development to support building a data-driven organization
- build community for clinical data science across NYU Langone
- facilitate external collaborations
- vet commercial AI-based technologies for implementation at NYU Langone
Learn more about our team and specific analyses.
Generative AI
Generative AI is a new type of AI that can create new content, such as images, videos, and text. Applications include ChatGPT, Google’s Bard, and Microsoft’s Bing, among others. These applications have potential uses that are now being explored by scientists and clinicians but require further development and careful consideration before use. This technology has the potential for transformative change in how NYU Langone delivers patient care, educates, conducts research, and manages operations.
The Predictive Analytics Unit is the collaboration hub for governance, expertise, and shared tools to maximize the effectiveness of generative AI customized for the NYU Langone community.
NYU Langone has partnered with Microsoft and other vendors to leverage these technologies in a private, safe, and secure manner.
Access NYU Langone’s Dedicated Generative AI Private Environment
As a member of the NYU Langone community, if you are interested in engaging with generative AI to support a specific project related to patient care, education, research, or operations, please submit a consultative request for access or a request to be granted exploration access for potential use cases.
Please keep in mind the following factors:
- Access to NYU Langone’s generative AI private environment occurs on a rolling basis to ensure a great experience for all users.
- Access is prioritized based on whether you are part of an innovation/research project, mentored exploration project, or exploration project.
- Users are notified by email regarding approval of access and must sign a data use agreement and are required to review further information on accessing our managed instance.
If you have a non-sensitive and non-business use for generative AI, please use the public ChatGPT. Please remember that it is of utmost importance that any use of generative AI applications be in full compliance with existing policies and the law:
- You must not use any generative AI application in connection with or to generate your clinical documentation, such as notes included in the medical record or letters.
- You must not use any generative AI application with any protected health information, even if de-identified, or any other legally protected information.
- You must not use any generative AI application with any clinical or human subjects research data, even if de-identified.
- Do not disclose to any generative AI application any confidential business information.
- Do not permit any generative AI application to record or otherwise upload recordings of internal meetings or other non-public NYU Langone activities.
- Do not rely on any generative AI applications or rely upon results from any generative AI application in your work at NYU Langone Health. You are responsible for independently verifying any generative AI content.
If you have any questions or concerns about this guidance, please contact us at Generative.AI@NYULangone.org.
Generative AI Events
The Predictive Analytics Unit in the MCIT Department of Health Informatics hosts events to educate the NYU Langone community and the public about the application of generative AI in healthcare.
Generative AI at NYU Langone: Our Future with ChatGPT, GPT, Bard, Bing, and Others
Dr. Aphinyanaphongs, Marc M. Triola, MD, and other members of the Predictive Analytics Unit team hosted a generative AI webinar (Kerberos ID and password required) to explore the following topics: what is generative AI, why is it potentially transformative, and how can it be used at NYU Langone, as well as ethical implications of generative AI and appropriate use.
Generative AI Resources
For more information on generative AI, please see Additional Educational Resources for AI in Healthcare and NYU Langone Health AI Studio—Video Tutorials.
Selected Predictive Analytics and Machine Learning Publications
Hammond R … Elbel B. Predicting childhood obesity using electronic health records and publicly available data. PLoS One. 2019. DOI.
Coudray N … Tsirigos A. Classification and mutation prediction from non-small cell lung cancer histopathology images using deep learning. Nat Med. 2018. DOI.
Liu J, Zhang Z, and Razavian N. Deep EHR: Chronic disease prediction using medical notes. Proceedings of the 3rd Machine Learning for Healthcare Conference. 2018. 85:440–464.
Aphinyanaphongs Y. Big data analyses in health and opportunities for research in radiology. Semin Musculoskelet Radiol. 2017. DOI.
Blecker S … Katz SD. Early identification of patients with acute decompensated heart failure. J Card Fail. 2017. DOI.
Blecker S … Sontag D. Comparison of approaches for heart failure case identification from electronic health record data. JAMA Cardiology. 2016. DOI.
More Information and Resources
Learn more about the Predictive Analytics Unit and find follow-up resources (Kerberos ID required to log in).